R, Notes for Professionals.
Фрагмент из книги:
In R, data objects are manipulated using named data structures. The names of the objects might be called "variables" although that term does not have a specific meaning in the official R documentation. R names are case sensitive and may contain alphanumeric characters(a-z,A-z,0-9), the dot/period(.) and underscore(_). To create names for the data structures, we have to follow the following rules.
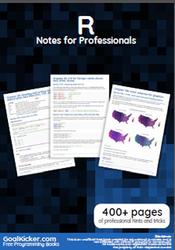
Types of data structures.
There are no scalar data types in R. Vectors of length-one act like scalars.
• Vectors: Atomic vectors must be sequence of same-class objects.: a sequence of numbers, or a sequence of logicals or a sequence of characters, v <- c(2, 3, 7, 10), v2 <- c("a", "b", "c") are both vectors.
• Matrices: A matrix of numbers, logical or characters, a <- matrix(data = c(1, 2, 3, 4, 5, 6, 7, 8, 9, 10, 11, 12), nrov/ = 4, ncol = 3, byrow = F). Like vectors, matrix must be made of same-class elements. To extract elements from a matrix rows and columns must be specified: a[ 1,2] returns [1 ] 5 that is the element on the first row, second column.
• Lists: concatenation of different elements mylist <- list (course = 'stat', date = '04/07/2009', num_isc = 7, num_cons = 6, num_mat = as.character(c(45020, 45679, 46789, 43126, 42345, 47568, 45674)), results = c(30, 19, 29, NA, 25, 26 ,27) ). Extracting elements from a list can be done by name (if the list is named) or by index. In the given example mylistSresults and mylist [ [6] ] obtains the same element. Warning: if you try mylist [6], R won't give you an error, but it extract the result as a list. While mylist[ [6] ] [2] is permitted (it gives you 19), mylist[6] [2] gives you an error.
• data.frame: object with columns that are vectors of equal length, but (possibly) different types. They are not matrices, exam <- data.frame(matr = as.character(c(45020, 45679, 46789, 43126, 42345, 47568, 45674)), res_S = c(30, 19, 29, NA, 25, 26, 27), res_0 = c(3, 3, 1, NA, 3, 2, NA), res.TOT = c(30,22,30,NA,28,28,27)). Columns can be read by name examSmatr, exam[, 'matr' ] or by index exam[1 ], exam[, 1 ]. Rows can also be read by name exam[' rowname', ] or index exam[1, ]. Dataframes are actually just lists with a particular structure (rownames-attribute and equal length components)
Contents.
About.
Chapter 1: Getting started with R Language.
Chapter 2: Variables.
Chapter 3: Arithmetic Operators.
Chapter 4: Matrices.
Chapter 5: Formula.
Chapter 6: Reading and writing strings.
Chapter 7: String manipulation with stringi package.
Chapter 8: Classes.
Chapter 9: Lists.
Chapter 10: Hashmaps.
Chapter 11: Creating vectors.
Chapter 12: Date and Time.
Chapter 13: The Date class.
Chapter 14: Date-time classes (POSIXct and POSIXlt).
Chapter 15: The character class.
Chapter 16: Numeric classes and storage modes.
Chapter 17: The logical class.
Chapter 18: Data frames.
Chapter 19: Split function.
Chapter 20: Reading and writing tabular data in plain-text files (CSV, TSV, etc.).
Chapter 21: Pipe operators (%>% and others).
Chapter 22: Linear Models (Regression).
Chapter 23: data.table.
Chapter 24: Pivot and unpivot with data.table.
Chapter 25: Bar Chart.
Chapter 26: Base Plotting.
Chapter 27: boxplot.
Chapter 28: ggplot2.
Chapter 29: Factors.
Chapter 30: Pattern Matching and Replacement.
Chapter 31: Run-length encoding.
Chapter 32: Speeding up tough-to-vectorize code.
Chapter 33: Introduction to Geographical Maps.
Chapter 34: Set operations.
Chapter 35: tidyverse.
Chapter 36: Rcpp.
Chapter 37: Random Numbers Generator.
Chapter 38: Parallel processing.
Chapter 39: Subsetting.
Chapter 40: Debugging.
Chapter 41: Installing packages.
Chapter 42: Inspecting packages.
Chapter 43: Creating packages with devtools.
Chapter 44: Using pipe assignment in your own package %<>%: How to?.
Chapter 45: Arima Models.
Chapter 46: Distribution Functions.
Chapter 47: Shiny.
Chapter 48: spatial analysis.
Chapter 49: sqldf.
Chapter 50: Code profiling.
Chapter 51: Control flow structures.
Chapter 52: Column wise operation.
Chapter 53: JSON.
Chapter 54: RODBC.
Chapter 55: lubridate.
Chapter 56: Time Series and Forecasting.
Chapter 57: strsplit function.
Chapter 58: Web scraping and parsing.
Chapter 59: Generalized linear models.
Chapter 60: Reshaping data between long and wide forms.
Chapter 61: RMarkdown and knitr presentation.
Chapter 62: Scope of variables.
Chapter 63: Performing a Permutation Test.
Chapter 64: xgboost.
Chapter 65: R code vectorization best practices.
Chapter 66: Missing values.
Chapter 67: Hierarchical Linear Modeling.
Chapter 68: *apply family of functions (functionals).
Chapter 69: Text mining.
Chapter 70: ANOVA.
Credits.
You may also like.
Бесплатно скачать электронную книгу в удобном формате, смотреть и читать:
Скачать книгу R, Notes for Professionals - fileskachat.com, быстрое и бесплатное скачивание.
Скачать pdf
Ниже можно купить эту книгу, если она есть в продаже, и похожие книги по лучшей цене со скидкой с доставкой по всей России.Купить книги
Скачать - pdf - Яндекс.Диск.
Теги: учебник по программированию :: программирование